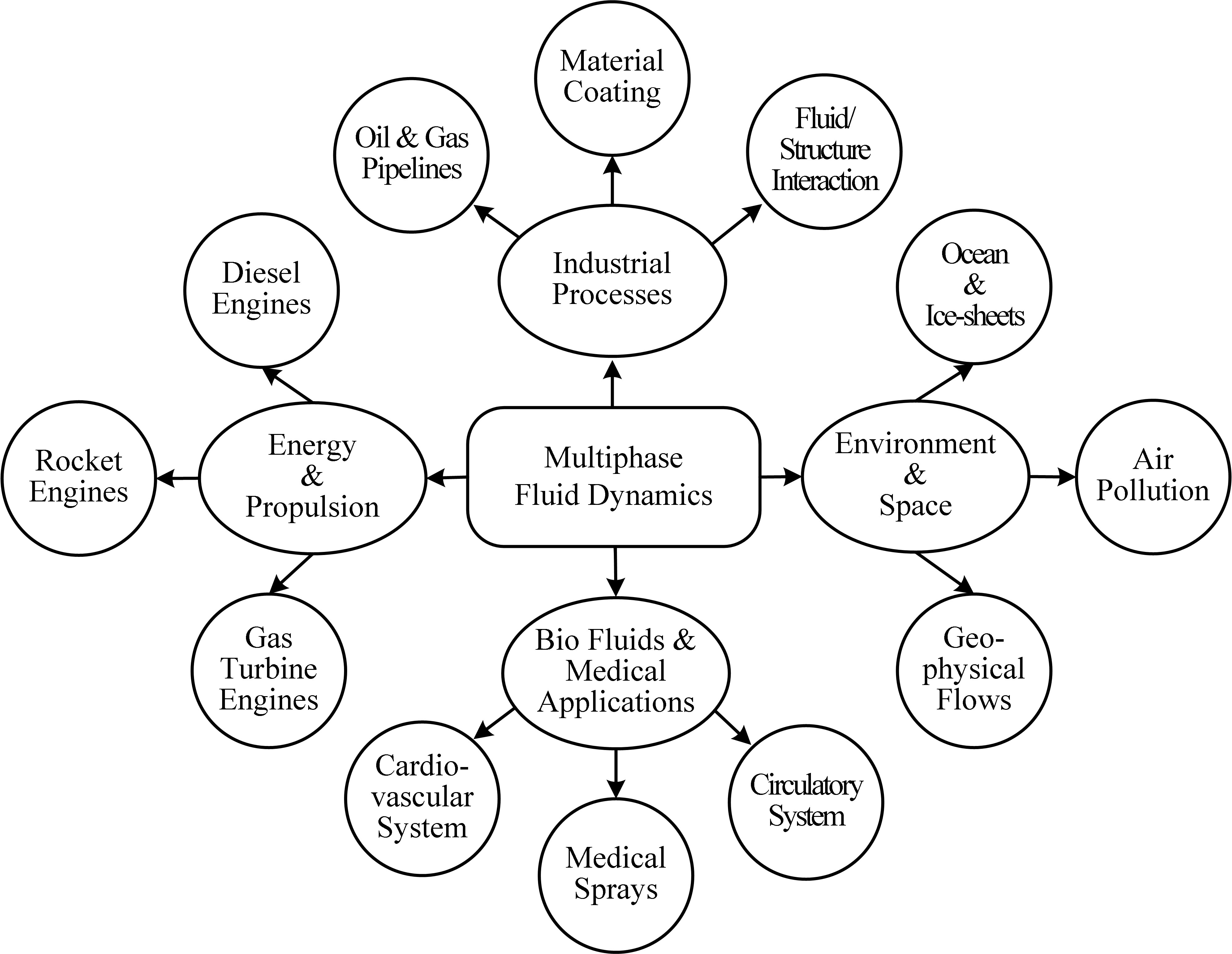
About
About the lab and the PI, click to read more.
We develop machine learning algorithms to predict spatiotemporal behaviors of turbulent, non-reacting and reacting multiphase flows to facilitate design space explorations of practical engineering devices. Our algorithms are not limited to predicting specific global quantities, but we predict all the details including interfacial instabilities, turbulent statistics, and spatiotemporal evolution of all relevant quantities.
Please right click on the video, choose show controls, and click the play button to play the videos.Comparison of diesel jet inejction and atomization predicted by machine learning (right) with DNS-based computations (left). | Comparison of flow dynamics when air flows over a circular cylinder predicted by machine learning (right) with DNS-based computations (left). |
About the lab and the PI, click to read more.
High-fidelity simulations of primary and secondary atomization of liquid fuels. Click here to read more..
Spatiotemporal prediction of non-reacting and reacting multiphase flows using machine learning. Click here to read more.
Decomposition and ignition of liquid fuels. Click here to read more.
Combustion dynamics of reacting gaseous and liquid fuels. Click here to read more.
Read our publications here.
Click here to read more about the high-performance computing software and hardware facilities.
Click here to read about recent events in the group
Main page for the ONR program. Click here to read more.
Click here to locate us on the map and other contact information.